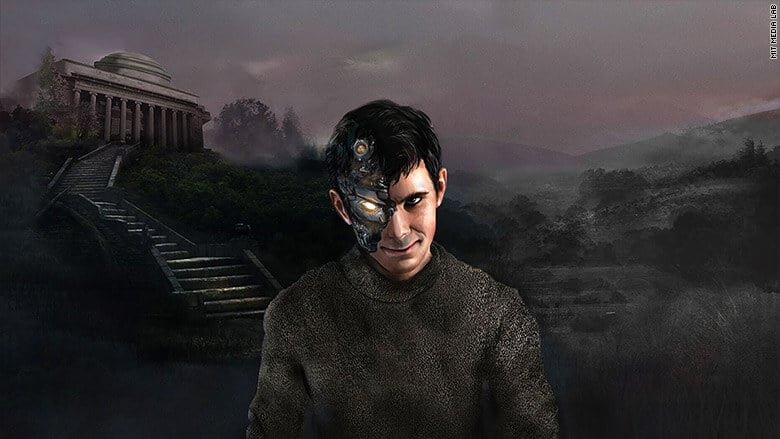
Data is, no doubt, an irreplaceable part in today’s technological world. Just by reading this, you’re producing a stream of data that’ll be analyzed for marketing, sales, etc. But somewhere along the way, the data might become biased and create problems. Biased data is one of the biggest overlooked dangers in every industry. Data is in theory neutral because it doesn’t hold opinions, just details. However, anyone handling the data can easily translate it to have a different meaning. Everything from data collection to interpretation is in the hands of human bias. And sometimes, it’s the data itself that’s dangerous. Sure, technology like artificial intelligence and machine learning have made great strides, but biased data is disruptive and can have disastrous consequences.
Disasters: Human Prejudice or Missing Data?
Biased data is data that is inaccurately skewed or intentionally focuses on specific for a calculated outcome. Artificial and machine learning have allowed us to create autonomous software. All AI needs is the right set of algorithms. However, no matter how independent data and AI are, we are still flawed. The algorithms and data that are put into the program or software can unintentionally carry strong bias. Algorithmic bias often arises from the code that we write. What you choose to include or exclude can drastically change the results. Microsoft previously launched an AI social media account named Tay designed to behave like a young millennial and learn from interactions. This quickly turned into a racist, sexist disaster after social media users took advantage of Tay’s mimicry. Was there an algorithm that would avoid inflammatory speech? Guess not, but there should have been. Data was certainly “missing” when designing Tay which left it at the mercy of internet trolls and bigots.
To prove just how strong biased data can be, MIT’s Media Lab created Norman, the first AI psychopath. The team fed Norman very dark and gruesome content from a very inappropriate subReddit, thus creating a psychopath. The resulting personality was tested with an ink blot test. While the ink blot test is subjective, Norman only communicated gruesome content and nothing else because that’s the data he was fed. Both Tay and Norman learned from biased data and it didn’t take much for either to become unhinged. AI personas are contained to their digital worlds, but discriminatory data in the real world has even more dangerous repercussions.
Oversight
Underlying prejudices produce flawed algorithms that create intense biases. All the terrible -ism’s are so deeply ingrained that they’re easily translated into the data. Most of medical data derived from clinical cases and studies only focus on men. That data of course is accurate but the blatant sexism is overlooked. Well, why don’t they just conduct more studies with women? The gender bias is so strong that women are simply taken less seriously as patients. Studies today still draw fewer female subjects and some clinical information is that of past male subjects. Women continue to be overlooked and misdiagnosed because of the original bias, which can lead to death. Women in the medical field have fewer opportunities to advance in the workplace. Overcoming prejudiced bias takes more than just analyzing data, but it can be an effective start.
Racism is so strong that (like the criminal justice system) technology is corrupted. Some courtrooms in Florida use risk assessment to consider how likely someone is to commit another a crime. Northpointe’s COMPAS software uses algorithms to calculate the likelihood of defendants reoffending, labelling them on a scale of low to high risk.
The algorithm should logically consider factors such as past convictions and aid the decision for release or sentencing. But results indicated the opposite. Propublica analyzed cases and the software’s predictions to find that only 20% of people predicted reoffended. Why was it so inaccurate? Human prejudice had taken the form of courtroom software. Propublica discovered that black defendants were twice as likely to be labelled as dangerous than white defendants, even when there was no logical evidence. Who do think would have a higher risk assessment? Someone with multiple armed robbery convictions caught stealing again or someone who took a child’s scooter. The COMPAS software predicted Borden, a young black 18 year old girl, was at a high risk of committing another crime in the next two years. Prater on the other hand, a 42 year old white man was a low risk. Prater ended up serving time on another robbery charge, just like his past convictions. It seems fairly obvious who’s at high risk, so how did COMPAS get it wrong? Simple, the creators made it that way. COMPAS, like other “intelligent” software, simply mimicked what it was taught (Northpointe refuses to divulge the algorithms).
How do you avoid bias?
You don’t have to create AI to see consequences of biased data. It can happen with a website, an app, and anything else that relies on data. And no matter how deeply you delve into data, there’s always something to look out for. Going too far into the details and you might lose sight of the big picture. Look at it at a glance, you’ll miss all the possible scenarios and circumstances that create a narrative. Ignoring certain details can also create bias, regardless of intent.
There’s no real answer to being objective, you can only be careful. Data analysis is still one of the greatest tools you have. Testing and experimenting can unearth insights critical to performance (and avoiding disastrous bias). So, take the time to understand the data even if it means reanalyzing.