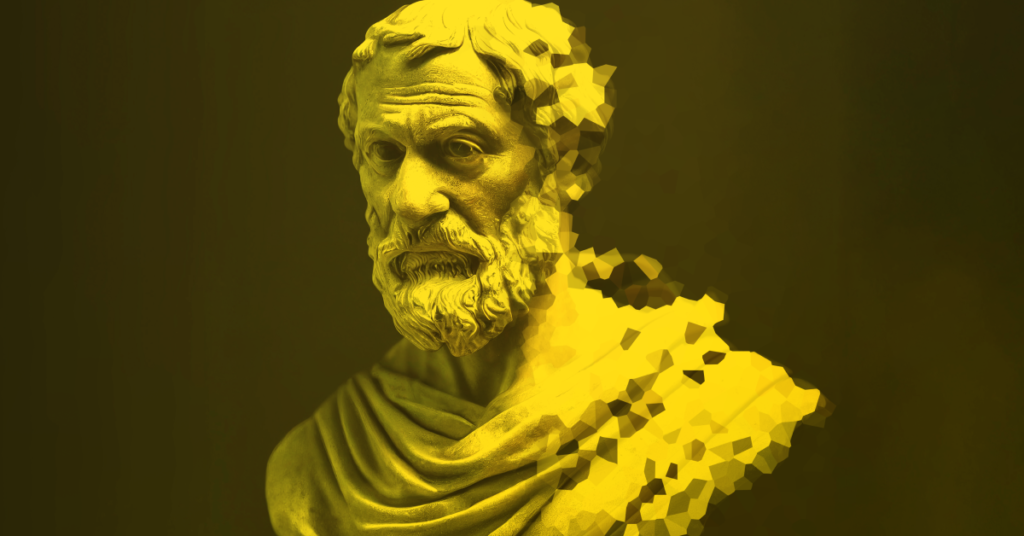
As digital product owners and data analysts alike, it’s our responsibility to understand and avoid common data fallacies that lead to faulty assumptions, inaccurate conclusions, and even bad decision-making. Let’s take a look at some of these fallacies so we can all make more informed decisions based on trustworthy data.
The Base Rate Fallacy
The base rate fallacy refers to the tendency for people to overlook the overall picture in favor of focusing on details. This is a consequence of overconfidence bias, which is when individuals overly rely on their past experiences and underestimate their ability to predict future outcomes with accuracy. To illustrate this concept, consider the following example: someone might assert that, “All toupees look fake. I’ve never seen a good toupee.” This is an example of neglecting the base rate because if I had seen good toupees, I wouldn’t know it. I would have thought they were real hair and they would go unnoticed, thereby not included in the dataset from which I’m basing my claim.
The Gambler’s Fallacy
Also known as the Monte Carlo fallacy, this fallacy occurs when someone assumes that future events are affected by past events in a patterned way. For example, if a coin has been flipped heads five times in a row, someone might assume that the sixth flip will land on tails—regardless of the actual probabilities of each flip. The gambler’s fallacy is dangerous because it leads people to think they can predict outcomes with certainty when there is no evidence to support such claims.
False Correlation
False correlation occurs when two variables appear to be related but have no causal relationship whatsoever. For example, let’s say you’re analyzing sales data for camping equipment over time and you find that sales of these products tend to peak during summer months. If you overlay this data with weather data showing that summer months were also the times of peak rainfall, you might assume that rainy weather causes people to buy more camping equipment—but upon further inspection you realize that the increased demand actually comes from people gearing up for summer outdoor activities. In other words, the correlation between high rainfall and increased demand was simply an illusion; there was no real causal relationship between them.
Confirmation Bias
Confirmation bias refers to our tendency to focus on information that confirms our existing beliefs while discounting information that contradicts them. This type of bias can lead us astray by shaping our interpretations of data in such a way so as to fit neatly into whatever narrative we want it to tell us—even if it goes against reality or facts at hand. It’s important to remain mindful of confirmation bias when analyzing data so as not to draw false conclusions due to preconceived notions or expectations about what the results should be.
Conclusion:
Data analysis is an integral part of any digital product owner or data analyst role; therefore, it is important for us all to understand how common logical fallacies work so we can avoid them in our data practice and make better decisions based on factual evidence rather than incorrect assumptions or biases. By understanding these common fallacies–such as the base rate fallacy, gambler’s fallacy, false correlation, and confirmation bias–we can overcome them and make sure our decisions are soundly rooted in reality rather than wishful thinking or misleading information.