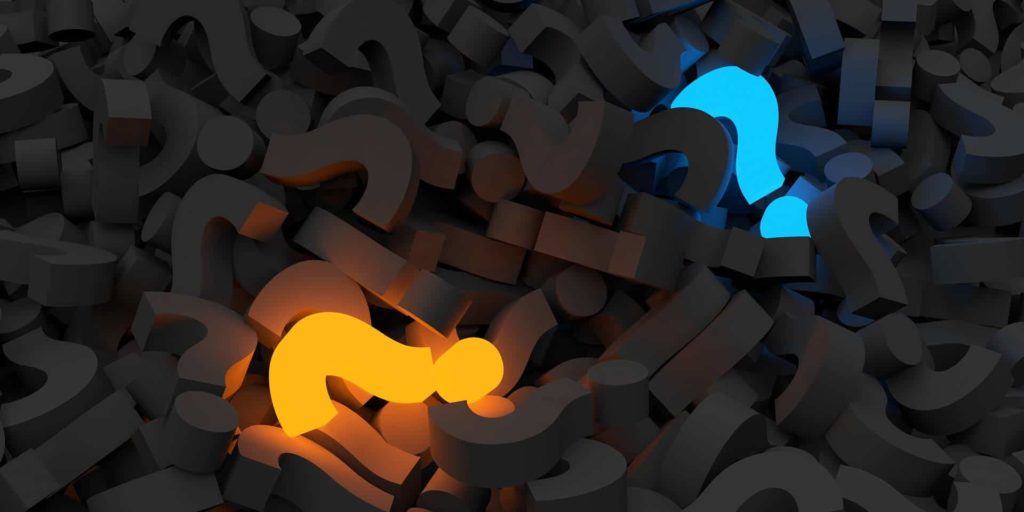
Data analysis opens up new insights for better decision making, but can be severely limited by the kind of analytics . Simply having lots of data and expecting results is never enough. You need to truly understand the data and the best way to represent it to actually gain meaningful insights.
If you want to extract value from data, you must first appropriately analyze it. Not all data sets should be treated the same way. Exploratory and Explanatory data analytics are 2 ways to initially handle raw data and used differently. Yes, that’s right. There are different types of analytics that provide deeper understanding for different integrations. You can go descriptive, predictive, or prescriptive (or a combination) for your desired outcome.
What does each type of analytics do?
Exploratory data analytics refers to the various ways to explore data. When you have a raw data set, it won’t provide any insight until you start to organize it. This can be some kind of readable format like an excel spreadsheet or, depending on your data, a complex visual model that visualizes data points. An in-depth exploration means structuring and visualizing data so you can identify patterns, outliers, anomalies, and other factors. What insights does one visualization show that another doesn’t? Why is this data point so different from the others? What do I need from this analysis for implementation? This is the first investigation to crack open clues and evidence. Whether it’s identifying crucial categories or building accuracy, exploring your data is a must. It sounds simple enough, but using different visualizations, tables, charts, or any other form takes time and requires a keen eye for detail. Especially important to data science projects, an exploratory analysis helps data scientists understand the data so they can create accurate algorithms and deeper understanding before implementation.
Explanatory data analytics focuses on all the parts of context, mainly the why and how. An outcome can be statistically calculated, modeled, or visualized to tell you the likelihood of certain events based on preconceived variables. However, it doesn’t convey the necessary and effective actions you should take in real life. Often used in marketing, explanatory analytics helps explain circumstances like why customers aren’t buying or a campaign is so successful and how to act on those insights. Should you keep using the same campaign only because it’s popular on social media OR analyze engagement and sales connected to specific demographics to optimize future performance? Connecting data insights to real life produces more effective context for better decision making and ROI.
Explore or explain?
Analyzing any large data set isn’t easy and isn’t the same for everyone. But everyone needs realistic insights to improve performance. The bottom line is any initial approach to data requires care and scrutiny in order to extract quality insights. There’s no one absolute roadmap for better insight so don’t be afraid to fully explore and explain your data.